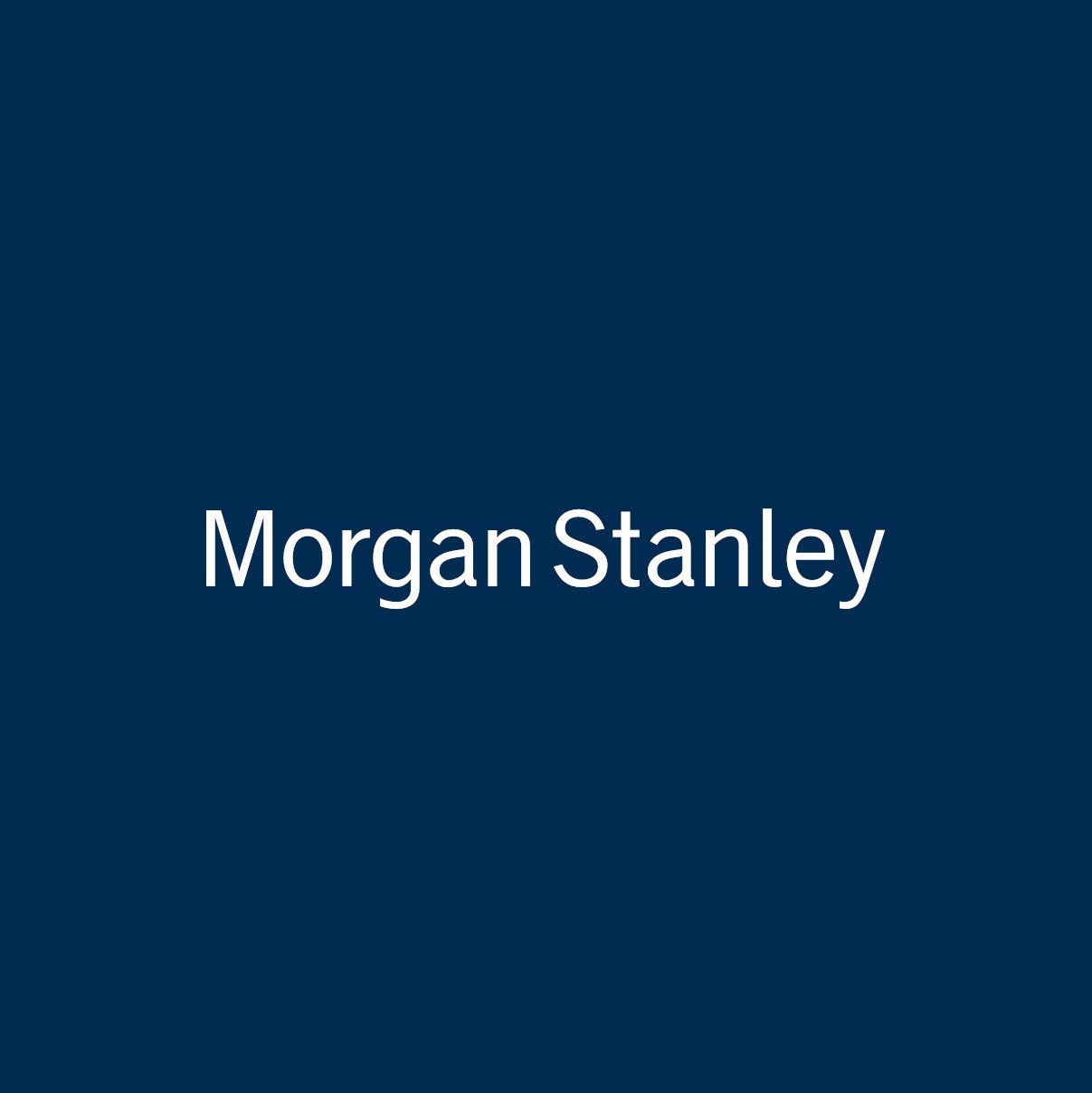
Financial Consulting and Research
Overview
Prior to my first full-time role at Party Round, I completed two part-time roles at VC firms and was a summer analyst at Morgan Stanley, and managed freelance financial consulting projects.
At MS, I collaborated with a small team and designed and proposed stress test improvements to adjust to COVID-19 risk factors by analyzing 2008 Global Economic Crisis fundamental risk safeguards.
I was grateful for receiving a full-time return offer from MS, but I ultimately decided to part ways from my corporate financial journey as I wanted to go further with building tools at startups.
I started freelance consulting for several Series A and early stage companies and built bespoke financial models from scratch for their fundraising and budgeting needs.
Highlights
JuneShine — managed investor and shareholder communications for $500k Series A syndicate including pitch deck and memo
Hopscotch — built pre-seed and seed model with revenue planning and presented to investors. Hopscotch raised a 3.6M seed round. They recently raised a $10M Series A
Produced Confidential Information Memorandums, pitch books, financial models via DCF and Sensitivity Analysis, and market updates for senior bankers and clients detailing competitive landscape, industry, defensibility, and growth opportunities
Presented models and analysis to investors on behalf of the client for fundraising

[Publication] Startup Investment Decision Support: Application of Venture Capital Scorecards Using Machine Learning Approaches
I was curious about the merits of qualitative traits and trends for venture capital due diligence methods. I investigated and built a scorecard model to test these hypotheses using various machine learning models.
The article was peer-reviewed and published in the Special Issue Computational Modeling Approaches to Finance and Fintech Innovation from the Systems journal. I've included the publication summary and approaches in this portfolio to demonstrate my relevant research background. The full article is here.
Authors
First author
Sarah Bai
Co-author
Yijun Zhao
Problem
There is no standard method of venture capital due diligence or evaluation methods and every firm has their own thesis. VC firms may consider a wide range of quantitative and qualitative factors when evaluating an early-stage startup such as the industry sector, amount of funding, return on investment trends, management team, relevant qualifications of the founders, market potential, and competitive advantage.
Qualitative factors are more difficult to weigh and I aimed to learn how certain qualitative factors stacked up against the overall investment theses of certain firms.
Goals
Evaluate the weight of relevant qualitative factors for startups for a venture capital firm's investment thesis
Develop models to test which machine learning methods may be the most accurate in predicting investment decisions that align with specifc theses
Discover which qualitative characteristics may be more favorable or critical to consider for a specific firm
Value
Iterative application — this research method may be applied for most venture capital firms to ascertain influential evaluation trends
Diligence methods — this study's findings may help inform ways to improve an investment firm approach for integrating qualitative factors in decisions
Process
In order to implement iterative evaluation methods, I chose a model-based type of venture capital decision-making process. The venture capital scorecard method converts any quality or variable in evaluation into a quantifiable table.
To facilitate this research, a voluntary venture capital firm studied 100 known late-stage companies since there is more public information available, enough for the firm to hypothetically make an investment decision retroactively based on the knowns.
The firm quantitatively scored a set of characteristics for each company that the management team believed to be highly pertinent in making the investment decisions.
The 100 companies were carefully selected to constitute an equal number of favorable (i.e., “yes”) and unfavorable (i.e., “no”) instances, and thus engendering a binary classification task in the machine learning domain. The companies were also chosen to encompass a wide variety of industries and sizes.

The industry distribution of the 100 selected sample companies that the firm reviewed, ranked through the scorecard model, and provided a hypothetical investment decision
Method
We fed the data into a machine learning tool and chose six common ML methods to test the data with for predictability and trends.
Evidenced by a noticeable performance gap between the two classes on a balanced dataset, our experimental results suggest that it is easier to identify attractive investments (i.e., “yes” class) as compared to those unfavorable ones (i.e., “no” class).
Furthermore, with the additional feature selection technique, the models are not only capable of delivering improved predictive accuracies but also effective in reducing the performance discrepancy between the two classes for some models.
These tailored models can be insightful in providing guidance on what company traits could be weighed more in future investment decisions.

We covered multiple machine learning methods so that model results could have more solid weighting indication. Team management and strategic roadmap are among the most important qualitative considerations for this firm.
Outcome
We had several key insights that may actually be applicable to venture capital firm approaches to their investment thesis and due diligence process.
1. Our study provides convincing evidence that machine learning models can be adapted to support investment decisions for VC firms.
2. We find that there should be a lower number of influential criteria to make efficient investment decisions that accurately reflect an investment thesis.
3. We also conclude that qualitative features are impactful in investment decision-making and suggest these features should be considered more than most basic quantitative features, depending on the firm.
4. If and when venture capital firms should demonstrate full conviction in the investment thesis, firms may follow relatively strict investment decisions due to the sway and high impact of fewer qualities.
5. Although our model is not perfect, our experimental results demonstrate effective predictive models that could serve as a Focus of Attention (FOA) tool for a VC firm.